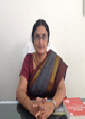
V Rama
Asst Professor in the ECE Dept., NIT, Warangal
Title: Early detection of Cardiac disorder using artificial intelligence
Biography
Biography: V Rama
Abstract
In the modern industrialized countries every year millions of people die due to cardiac disorders. India has highest incidence
of heart related diseases in the world. The WHO’s world statictics report 2016 shows that the life expectancy in India was
68.3 years. If no initiative is taken to check this most predictable and preventable among all chronic diseases, life expectancy
may further decrease. The main aim of this work is to enable the clinical doctors to diagnose cardiac disorders using computer
based analysis as the manual analysis is tedious and time consuming task. Early detection of cardiac disease is of paramount
importance for saving the life of patients. Electrocardiogram (ECG) is an important bio-medical signal representing the
electrical activity of the heart. A significant amount of work has been done in the past to identify cardiac disorders. All the
developed linear algorithms considered that the ECG signal as stationery. But on observing the fact that ECG is non-stationery.
It has been duly noted from Maedeh Kiani Sarkaleh (2012) paper in which the author has successfully classified 10 files,
which include both normal and two types of cardiac disorders and produced results with an accuracy of 96.5%. However, this
paper concentrates on developing novel feature extraction scheme using nonlinear signal processing technique and supervised
learning technique to improve classification accuracy of cardiac arrhythmias. The proposed system aims at classifying Normal
Sinus Rhythm (NSR) and 4 types of Cardiac arrhythmias-Sudden Cardiac Arrest (SCA), Ventricular Tachycardia(VT),
Ventricular Fibrillation(VF) and Cardiac Ischemia(CI). Daubechies wavelet transform (db4) 6-level decomposition technique
is used for extraction of ECG features. Finally these features are fed to Multi-Layer Perceptron Neural Network classifier.
The classification accuracy obtained is 100% and its computation time is only 0.28 seconds. Selection of optimum number of
features is essential to improve classification accuracy. The feasibility of all the above proposed method has been tested using
benchmarked MIT-BIH database and the performance of MLP classifier has been evaluated in Waikato Environment for
Knowledge Analysis.